A Family Affair: Implications for Streaming Service Recommendations
It’s now standard practice for video streaming services to include personalised recommendations to aid discovery and viewing choice. However, viewing often switches between a solo and group activity, and recommendations mechanisms typically don’t reflect this. AI helps, but this shift in context is too profound to ignore.
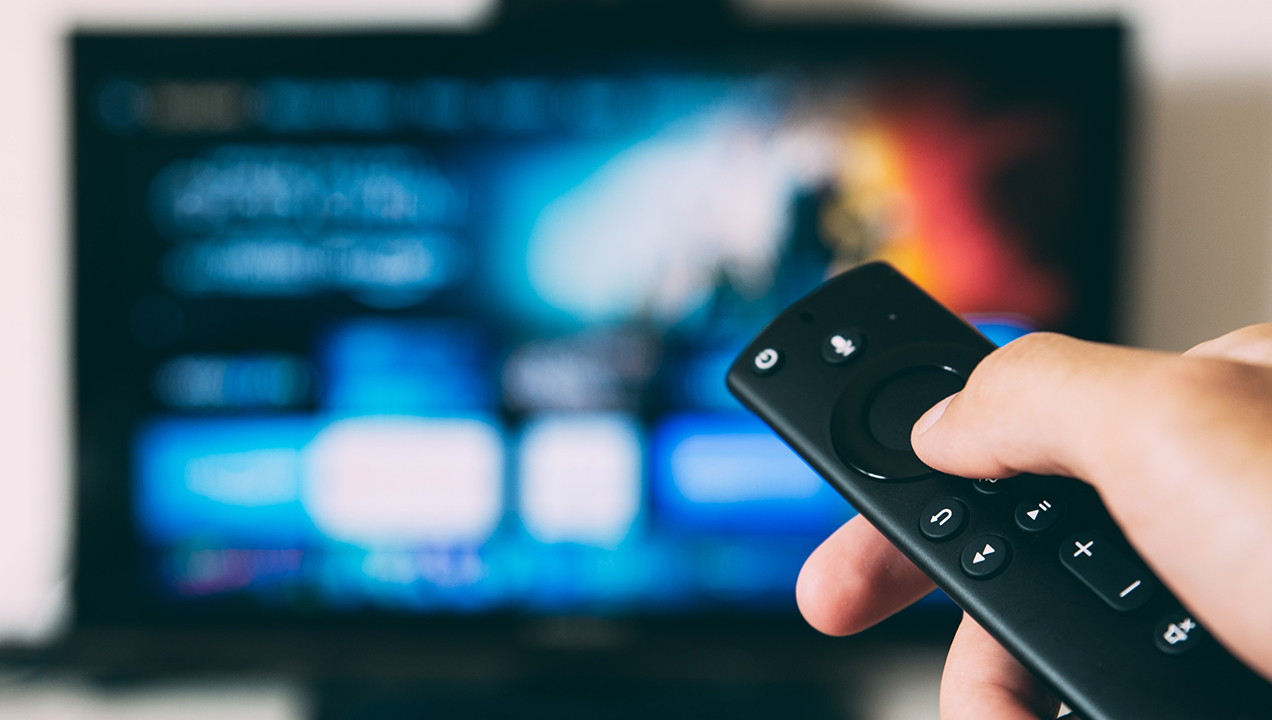
Photo Credit: Glenn Carstens-Peters
Personalisation, and viewing recommendations in particular, are now expected within streaming services. Their value in supporting viewers was demonstrated during 2019 by TiVO who reported that “Providers that use personalisation technology like the Personalised Content Discovery platform, churn up to three times less than providers who manually merchandise their content.” So recommendations are clearly here to stay, but they’re currently a one-size fits all solution.
Viewing choices on TV often involve compromise
We’ve found that programme choices do vary by device. Mobile phones and tablets are more suited to solo viewing, both due to size and the context they’re used within, so singular personalised recommendations are a strong fit.
However, this begins to break down when viewing is on the TV, as this is much more likely to be by more than one person. In fact, over 75% of all streamed video content consumed in the UK happens on a TV (Source: TouchPoints 2019, IPA, All adults). Viewing involving more than one person is a compromise, seeking the safe middle ground. It’s often led by one person, who makes viewing suggestions based on knowledge of individual tastes and perhaps even having spent time on sites such as Rotten Tomatoes finding good compromise watches.
Provider recommendations can thus be caught between highly singular viewing and compromise viewing needs. This also causes recommendation pollution, whereby the usefulness of recommendations is further degraded as compromise viewing influences future recommendations on their profiles. Parents with young children may find Peppa Pig or My Little Pony popping up within their recommendations alongside the latest episode of Vikings or Narcos for example. One approach is to provide individual products to manage familial viewing, such as Spotify Kids does for audio, but there are other options to do this within a singular product.
Just rely on the AI
The logic of AI is that recommendations get better with data, as more is known about viewing patterns, so naturally family friendly programming is likely to rise to the top at the times of day when joint viewing happens. Equally, singular pleasures such as reality TV may be more likely to appear on recommendations when viewed on mobile devices, as the AI has access to viewing platform information.
However, the AI doesn’t actually know you’re with other people, it can merely imply it from secondary factors such as programme watched, platform and time of day. There are a range of factors influencing viewing choice, with mood being key and this complexity is implicitly acknowledged by many recommendation features often having more than one panel and focus. The presence or absence of other people has a profound influence on choice and so justifies its own inclusion in the user interface for multi-person households.
Furthermore, the AI learns to improve the effectiveness of recommendations through a process of trial and error, as it learns which recommendations are accepted and rejected. The speed that this happens depends entirely on how frequently the recommendations panel is used, and there’s always a danger of users being turned off the feature entirely if the recommendations are just too inappropriate.
The presence or absence of other people has a profound influence on choice and so justifies its own inclusion in the user interface for multi-person households.
AI is powerful but it isn’t the total solution, it needs help. A really simple way to do this is to allow users to delete programmes from their recommendations, which can speed up the AI learning process.
Family accounts exist elsewhere
How can viewing recommendations do more to support the duality of singular/group viewing?
Well, the idea of household and individual accounts within products isn’t new. It’s supported by the likes of Apple, although the inherent complexity of family dynamics can make it difficult to do well, so it’s best to keep options as low in effort as possible.
Users can also create their own family profiles
Some households do create profiles for household and personal use, with the family profile (containing more compromise views) predominating on the TV. This is a potentially neat workaround which allows users to focus their compromise watches in one place, most likely on the TV. This places the greatest burden on the viewer, as it requires more profile switching at the start of viewing. It also involves other trade-offs, for example it may become slightly more difficult to continue watching a partially viewed programme on another device. This is unlikely to be practical in all but the most diverse households.
A family recommendations option
The other option is to incorporate a family recommendations panel in any profile on the home page, so it’s always available within a personal profile, but doesn’t predominate.
A similar concept exists within Spotify, with Family Mix, which draws on listening history from all members within a Family account. This also includes a mood qualifier to help listeners achieve a further level of depth to recommendations (we’ve found mood a key decision point for joint viewing) and feels like an interesting direction for combining personal and household viewing.
So what’s going to help families most?
AI is a powerful force for recommendations, but it’s unlikely to solve all the challenges of programme discovery itself. A multi-faceted approach to recommendations works well, but leading VOD services don’t include a choice for singular or group viewing in their UI.
Overall, the Spotify model of integrating group viewing feels like the most interesting as it’s easy to access without disrupting the rest of the UI. It also provides some additional granularity by including a mood qualifier, and a similar feature could be applied to TV.
In any instance we’d recommend aiding AI learning by allowing users to delete inappropriate recommendations, reducing recommendations pollution and increasing effectiveness. Effective tuning of auto-recommendations is always challenging, and combining these across a household is an added layer of complexity, but it does reflect how we watch VOD. Even as the number of devices proliferate, singular and joint viewing are likely to remain fundamental drivers for programme selection.
If you’d like to find out more about our work on streaming services, contact Simon Herd, or sign up for our newsletter below to receive future articles.